To The Brain and Back: Measuring The Brain’s Response to Continuous, Natural Speech
For decades, audiological practice and auditory neuroscience has benefitted from using electroencephalography (EEG) to measure brain responses evoked by speech sounds. Speech-evoked EEG responses show how hearing loss affects speech processing, how hearing aids provide speech perception benefits, and how the auditory system develops.1 The most common way to measure them is to repeatedly present single words or phonemes, usually hundreds of times, while recording the EEG. This is done because the speech-related brain response is small and largely hidden by the background activity of the EEG recording. The background noise is averaged out by averaging many repetitions together, leaving only the neural response that was time-locked to the onset of the speech sound. These averaging steps are the main idea behind the event-related potential (ERP) technique, and speech-evoked ERPs have been a mainstay in the research and clinical toolkit.
Though speech ERPs are useful, they do not reflect how speech is processed in everyday life. Real-life speech communication occurs when single words are embedded in sentences, ideas, and narratives. Speech interactions also occur in social settings, so the talkers' familiarity and social and cultural speech norms also add contextual richness. Context is important because a given word may be more predictable and more easily perceived based on words before or after, who is speaking, or how speech is intonated or timed. Listening to natural speech in context also engages cognitive systems, such as working memory, attention, and higher-level language processes that impinge upon speech perception. Everyday speech also happens with distractors and some background noise. Patients’ complaints about their speech listening ability likely arise in these contexts (e.g., listening to television, conversing with friends and family). If brain responses were measurable in these conditions, they would greatly complement knowledge derived from traditional speech ERP paradigms.
It is impractical to present patients with long, continuous speech passages hundreds of times, averaging them together to get a very long neural response as in the event-related potential technique. Fortunately in the last 15 years, new advancements have been made in EEG analysis that allow estimation of the brain’s response to continuous, natural speech, including stimuli such as audiobooks, films, and in-person conversation. There are many styles of these analyses referred to as speech tracking techniques,2,3 and here I will discuss a common one called the temporal response function (TRF).4 At a simple level, the TRF is a model or transformation that describes how continuous stimulation relates to continuous EEG activity. To measure a TRF, researchers will typically record the EEG while a person listens to natural, continuous speech. The audio signal is recorded alongside the EEG to be precisely aligned. Before the analysis starts, the researcher selects the characteristics or features of speech, most commonly the acoustic amplitude envelope, that they wish to map to the neural response.
Loosely, the TRF is calculated as a type of cross-correlation* between the audio signal and EEG. The brain response does not happen instantaneously as speech is heard, and there is usually 50-200 milliseconds before the cortical response occurs after speech onset. For this reason, cross-correlations are measured across a series of time differences or lags, so that the researcher can see how a change in the speech audio is associated with a change in the brain response many milliseconds later. This concept is made clearer in Figure 1, which shows a TRF to continuous speech presented in different background noise levels.
*The calculation for the TRF is more than a simple cross-correlation; for example, statistical regularization (e.g., ridge regression) is typical to create smoother models that are not overfit to the noise of the EEG.
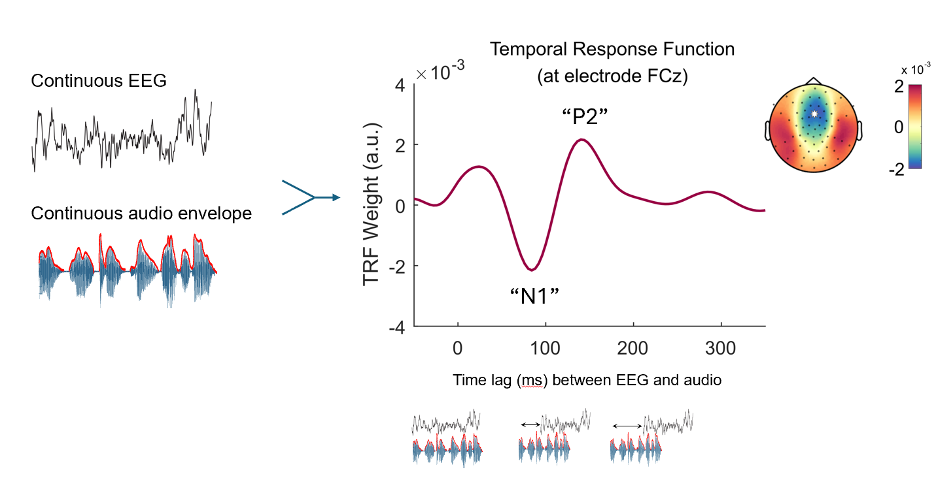
Researchers perform the TRF analysis on continuous EEG and some acoustic or linguistic characteristics of the speech signal, such as the audio envelope outlined in red. The TRF appears as a complex waveform representing the audio and EEG correspondence. The x-axis of the TRF plot on the right represents the time lag between the audio envelope and the EEG, representing how the EEG voltages change later after a change in the magnitude of the audio envelope. The y axis is the “weight” or coefficient representing the direction and strength of the relationship between the audio and EEG. TRFs can be computed for multiple EEG channels, and the TRF weights are shown at the N1 time lag across all 64 EEG channels in the multicoloured topographical plot.
Readers with EEG experience might note that the response itself resembles a typical event-related potential, showing positive (P1, P2) and negative peaks (N1) following the onset of speech. While ERPs show real time on the x-axis and voltage amplitude on the y-axis, the TRF plot shows a “weight” or coefficient between the brain response and the audio on the y-axis. Time lags, not real time, are on the x-axis. The N1 peak of the TRF†, highlighted in the figure, would indicate how a unit increase in the audio envelope correlates to the brain potential that occurs 100 ms later (i.e., a time lag of 100 ms). Here, the value is negative at 100 ms time lag, suggesting that the EEG creates a strong negative potential. Similarly, the P2 peak would reflect a positive increase in the brain potential at a 200 ms time lag. The topography of the TRF weights can also be viewed across the scalp if multiple EEG channels are recorded, as one would do with a traditional event-related potential. In short, TRFs provide a way to examine neural responses to speech using familiar metrics such as the time series of the neural signal and topographical maps. However, this response is derived from correlating the continuous EEG and continuous audio rather than looking at an average response to repeated, isolated speech events.
†A TRF's “N1” response should not be confused with the N1 of the event-related potential technique, but they may stem from similar neural origins.
Unique to the TRF method, the underlying model can either be computed to predict the brain signal from input speech (encoding models) or the original speech signal from the recorded brain response (decoding models). Figure 1 shows an encoding model (audio-to-speech). Still, decoding models are quite powerful because one can attempt to reconstruct the original speech signal from the brain response itself, which has many promising applications in engineering.5 The TRF technique has many other advantages for understanding speech processing that are difficult to achieve with the event-related potential technique. For example, you can compute TRFs to multiple streams of simultaneous speech, as a person would experience in a cocktail party-like setting.6 Additionally, TRFs can be estimated for both visual and auditory speech, which is useful for studying multisensory integration.7 Beyond using acoustic features of speech such as the audio envelope, TRFs can also be computed for higher-level language representations, such as phonemes, word entropy, and word surprisal. These latter representations provide insights into lexical and semantic processing across the brain surface. Therefore, from just one recording of a person’s EEG, it is possible to examine a hierarchy of speech perception and understanding, from acoustics to complex semantic meaning. Please see.8 for such examples.
How could TRFs or other speech-tracking techniques help audiological practice or hearing aid technology? One promising example is to use TRFs for auditory attention decoding. Because TRFs can be estimated to multiple, simultaneous talkers, future hearing devices may use small EEG sensors to pick up brain activity, which could steer hearing aid processors toward the speech a person wishes to attend based on the TRF. In my own research, I have used TRFs to study how cochlear implant users use selective attention to focus on one of two competing talkers.9 In addition, we have studied how background noise affects cochlear implant users’ neural speech responses while they listen to a television show, which also correlated with increased listening effort.10 TRFs could potentially serve as useful neural markers that track speech rehabilitation in CI users, or help the mapping process by finding configurations that yield robust neural responses. Hearing aid research has also used speech tracking to estimate speech intelligibility benefits from amplification.11 Importantly, patients and research participants also find continuous speech more enjoyable than repeated, isolated speech.
Whether or when clinical practice would adopt speech-tracking methods remains uncertain. However, if they were, speech-tracking methods could hold incredible promise for patient experiences and rehabilitation strategies. The audiologist may already be equipped with the necessary equipment. Brain responses suitable for this analysis can be measured with simple (e.g., 3-channel) EEG montages, and the response can be calculated by software. TRF measurement only requires minutes of recording, but 10 to 15 minutes of audio is most common in research.
References
- Martin, B.A., Tremblay, K.L. and Korczak, P., 2008. Speech evoked potentials: from the laboratory to the clinic. Ear and hearing, 29(3), pp.285-313.
- De Clercq P, Vanthornhout J, Vandermosten M, Francart T. Beyond linear neural envelope tracking: a mutual information approach. Journal of Neural Engineering. 2023 Mar 9;20(2):026007.
- De Cheveigné A, Wong DD, Di Liberto GM, Hjortkjær J, Slaney M, Lalor E. Decoding the auditory brain with canonical component analysis. NeuroImage. 2018 May 15;172:206-16.
- Crosse MJ, Di Liberto GM, Bednar A, Lalor EC. The multivariate temporal response function (mTRF) toolbox: a MATLAB toolbox for relating neural signals to continuous stimuli. Frontiers in human neuroscience. 2016 Nov 30;10:604.
- Wang L, Wu EX, Chen F. Contribution of RMS-Level-Based Speech Segments to Target Speech Decoding Under Noisy Conditions. Interspeech 2020 (pp. 121-124).
- Akram S, Simon JZ, Babadi B. Dynamic estimation of the auditory temporal response function from MEG in competing-speaker environments. IEEE Transactions on Biomedical Engineering. 2016 Nov 15;64(8):1896-905.
- Crosse MJ, Di Liberto GM, Lalor EC. Eye can hear clearly now: inverse effectiveness in natural audiovisual speech processing relies on long-term crossmodal temporal integration. Journal of Neuroscience. 2016 Sep 21;36(38):9888-95.
- Brodbeck C, Hong LE, Simon JZ. Rapid transformation from auditory to linguistic representations of continuous speech. Current Biology. 2018 Dec 17;28(24):3976-83.
- Paul BT, Uzelac M, Chan E, Dimitrijevic A. Poor early cortical differentiation of speech predicts perceptual difficulties of severely hearing-impaired listeners in multi-talker environments. Scientific Reports. 2020 Apr 9;10(1):6141.
- Xiu B, Paul BT, Chen JM, Le TN, Lin VY, Dimitrijevic A. Neural responses to naturalistic audiovisual speech are related to listening demand in cochlear implant users. Frontiers in Human Neuroscience. 2022 Nov 7;16:1043499.
- Van Hirtum T, Somers B, Dieudonné B, Verschueren E, Wouters J, Francart T. Neural envelope tracking predicts speech intelligibility and hearing aid benefit in children with hearing loss. Hearing Research. 2023 Nov 1;439:108893.