Mysteries of the Hearing Brain – Our Amazing Auditory Brains
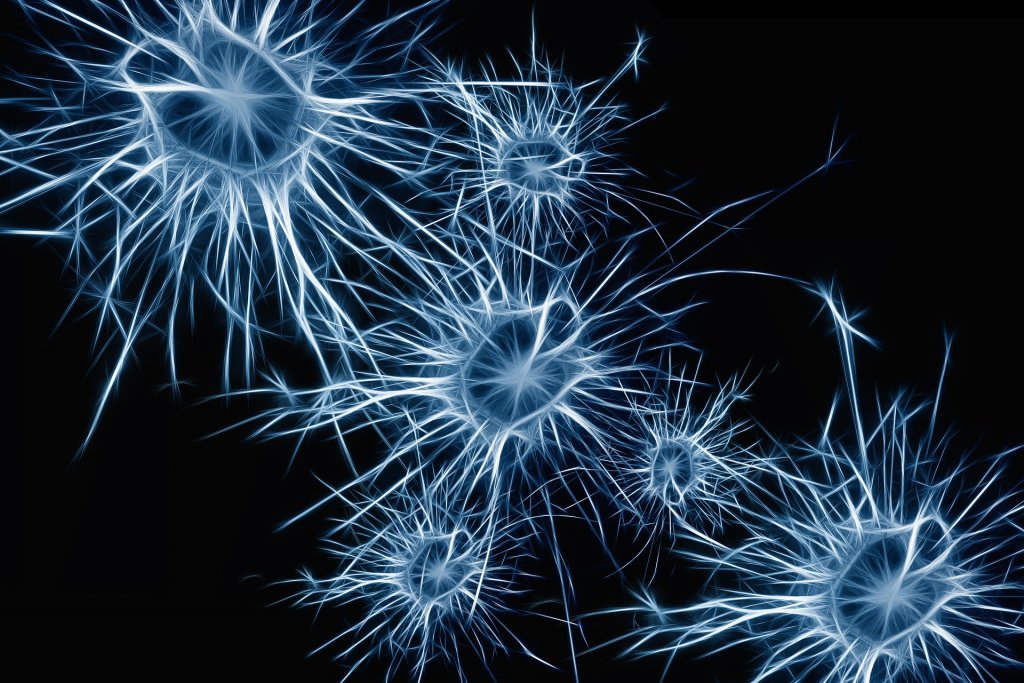
Our brains have an amazing capacity to reconstruct a distorted incoming auditory signal into a neural signal that resembles a “clean” or undistorted signal. In other words, if one were to compare the brain’s responses to speech presented in quiet and in noise, the brain’s response to speech presented in noise would be more similar to the original quiet speech signal rather than the noisy speech signal. This phenomenon was demonstrated in the brains of ferrets: spectrograms (representations of the neural signal’s frequency over time) constructed from responses to noisy or distorted signals resemble the original clean signal more than the noisy or distorted signals.1 These results demonstrate a neural mechanism that may underlie our ability to understand degraded and noisy signals.
In most situations, this transformation is seemingly effortless, at least in young adults. However, we know that older listeners struggle to understand speech in noisy or reverberant environments. One reason for the older listener’s speech understanding difficulty could be limitations in the older brain’s ability to compensate for a distorted or degraded speech signal. Vocoding is often used to simulate the degraded signal that is delivered to the cochlear implant listener. Vocoding preserves timing cues, such as amplitude changes over time, but it does not preserve the signal's frequency content. Therefore, an individual listening to a vocoded speech signal will need to rely on the speech signal's temporal cues to understand what is being said. Because aging is known to reduce the perception of temporal speech cues,2 older listeners’ speech understanding ability may be particularly affected by the degrading effects of vocoded signals.
A recent study evaluated the extent to which vocoding affected the perception and neural representation of temporal cues in younger and older listeners, specifically the silence duration cue that enables the listener to distinguish between words like DISH and DITCH (Anderson et al., 2020). If one inserts a silence duration of approximately 60 ms before the final /ʃ/ in DISH, the result is the perception of DITCH. This cue's perception was assessed by creating a seven-step continuum of silence duration varying from 0 to 60 ms in 10-ms increments. Eight-channel sine-wave vocoding was used to create a spectrally degraded version of this continuum. The unprocessed (clean) and vocoded continua were presented to young normal-hearing (YNH), older normal-hearing (ONH), and older hearing-impaired (OHI) listeners (15 in each group). The participants listened to randomly selected stimuli that corresponded to the seven steps on the continua and included both unprocessed and vocoded versions. Their task was to indicate whether they heard DISH or DITCH by selecting the appropriate response button on a graphical user interface. Figure 1 contrasts perceptual identification functions for the unprocessed and vocoded DISH-DITCH continua in the three listener groups. The results showed that vocoding did not affect perceptual performance in the younger listeners, but vocoding did affect the slope of the function in the older listeners, particularly in the older listeners with hearing loss. The slope was shallower in the vocoded vs. the unprocessed condition, indicating that the distinction between DISH and DITCH is less clear when the words are vocoded.
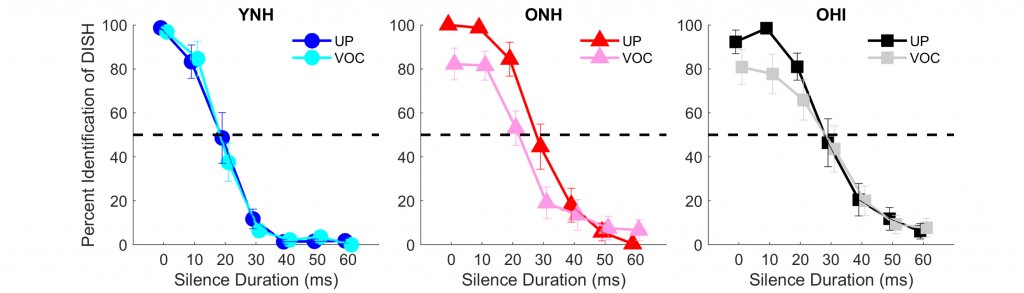
To evaluate the effects of vocoding on neural speech processing, frequency-following responses (FFRs) and cortical auditory-evoked potentials (CAEPs) were recorded to the endpoints (DISH and DITCH) of the unprocessed and vocoded continua. Figure 2 compares FFR magnitudes and CAEP waveforms in response to the DITCH stimulus presented in vocoded and unprocessed conditions to the three listeners groups. In contrast to the limited effects of vocoding on perception, there were substantial effects of vocoding on the FFR, with significant reductions in the magnitude of the fundamental frequency (100 Hz). In the CAEP waveforms, however, significant effects of vocoding were only noted in the ONH and OHI listeners but not the YNH listeners. The P1 peak, representing pre-perceptual detection of sound, was larger in response to the vocoded vs. the unprocessed DITCH stimulus. The larger amplitude may signify the greater effort needed to process the vocoded stimulus.
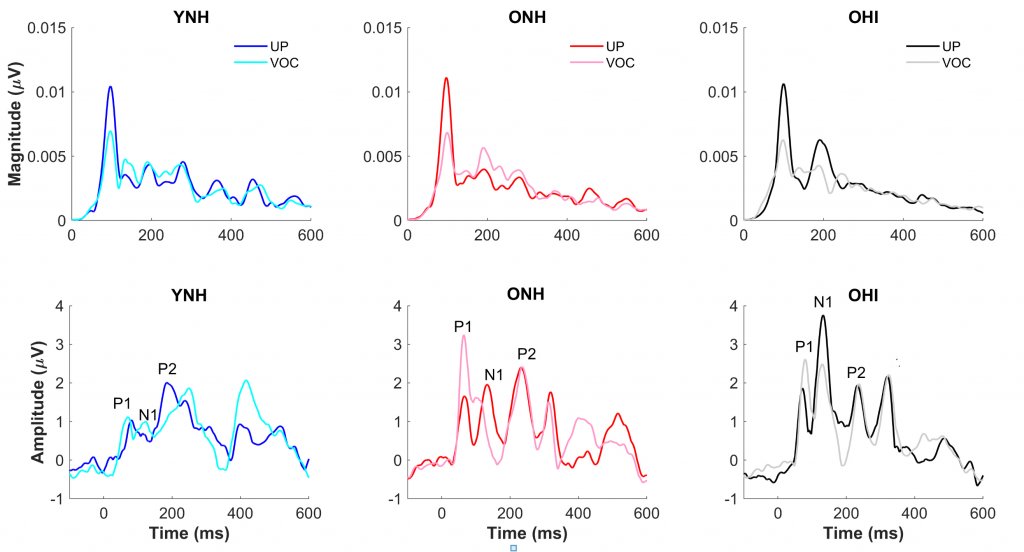
Overall, these results show that the cortex compensates for a degraded speech stimulus, perhaps in a similar manner to how ferret brains can recover a clean signal from a noisy or a reverberant signal. However, this compensation is not as robust in older listeners. These results suggest clinical implications for speech understanding outcomes in older cochlear implant (CI) users. Although the brains of CI users have a remarkable ability to convert incoming electrical pulse trains into meaningful speech signals, older users generally do not experience the same benefits in speech understanding as younger user.4,5 The compensation mechanism that is so effective in younger listeners may not be as effective in older listeners, leading to greater speech understanding difficulties. Given that older listeners rely on cognitive functions, such as working memory or attention, to improve speech understanding in difficult listening situations, it may be useful to develop auditory-cognitive training programs that target the older CI listener's needs.
References
- Mesgarani N., David SV, Fritz JB, Shamma SA. Mechanisms of noise robust representation of speech in primary auditory cortex. Proceedings of the National Academy of Sciences 2014;111:6792. 10.1073/pnas.1318017111.
- Roque L, Gaskins C, Gordon-Salant S, et al. Age effects on neural representation and perception of silence duration cues in speech. J Speech Lang Hear Res 2019; 62:1099–116, https://doi.org/10.1044/2018_jslhr-h-ascc7-18-0076.
- Anderson S, Roque L, Gaskins CR, et al. Age-related compensation mechanism revealed in the cortical representation of degraded speech. J Assoc Res Otolaryngol 2020.
https://doi.org/10.1007/s10162-020-00753-4. - Blamey P, Artieres F, Baskent D, et al. Factors affecting auditory performance of postlinguistically deaf adults using cochlear implants: an update with 2251 patients. Audio. Neurootol 2013;18:36–47, https://doi.org/10.1159/000343189.
- Sladen DP, Zappler A. Older and younger adult cochlear implant users: speech recognition in quiet and noise, quality of life, and music perception. Am J Audiol 2015;24:31–9. https://doi.org/10.1044/2014_aja-13-0066.